In today's manufacturing environments, maximizing productivity on the production floor, minimizing production losses that could result from machine and equipment failures, and planning maintenance times in advance for timely interventions are crucial. The training set developed to achieve these goals enables the control of machines and equipment using predictive methods to anticipate potential failures in advance. In the analysis, the initial operation of the machine is considered as its normal operating period. Anomaly detection is fundamentally a classification problem; in other words, it involves separating normal data from anomalous data. To successfully execute this process, it is essential to first collect data that is considered normal. Using this method, the aim is to identify anomalies that may occur later by initially accepting data obtained when the equipment starts operating as normal. Therefore, the predictive maintenance training set assists in minimizing production losses by planning maintenance times in advance and intervening promptly.
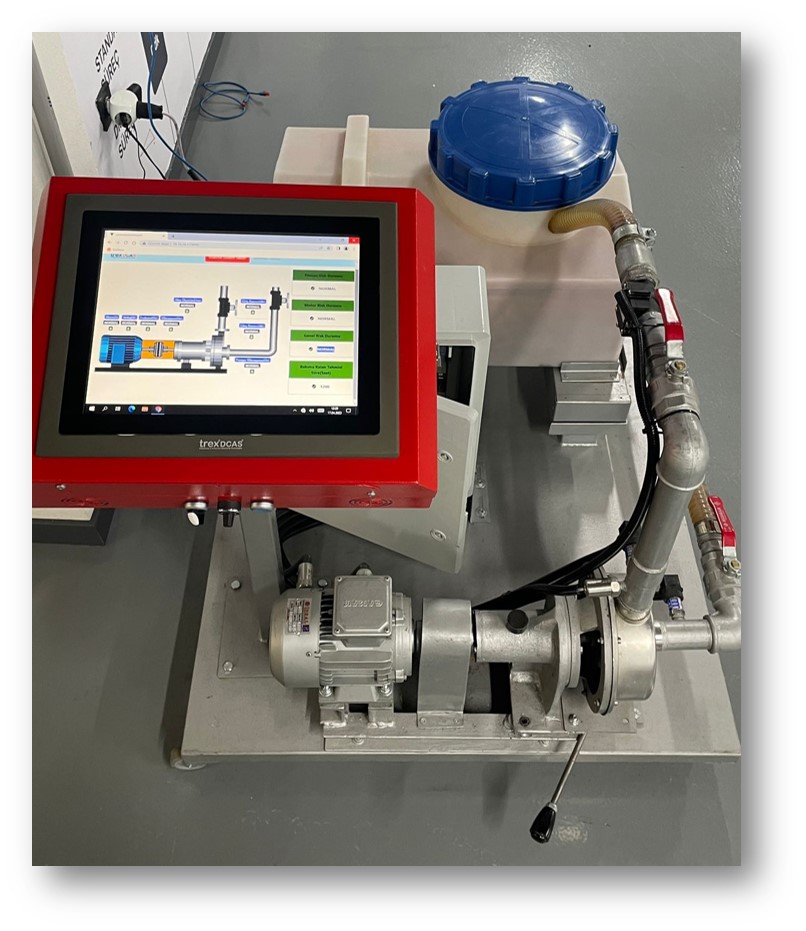